18. The future of healthcare – Keep people healthy with innovative health appliances and technologies
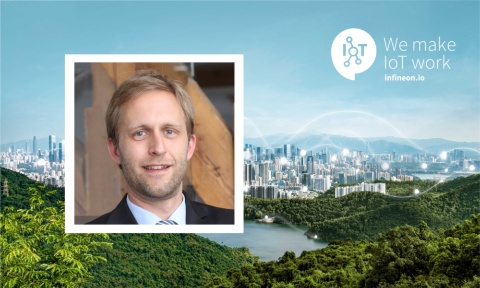
There has been a lot of discussion about the importance of good health and well-being, especially as the world population rises and at the same time life expectancy is increasing. Healthcare increasingly places great emphasis on enhancing well-being to prevent illness rather than sporadically treating acute illnesses. With smart electronic devices, either worn on the body or even installed at home, it is possible to measure people's fitness and health status and trigger appropriate actions. Here, data mining, algorithms, machine learning and artificial intelligence play a central role. This is what we want to talk about with our guest. Professor Björn Eskofier is heading the Machine Learning and Data Analytics Lab of the Friedrich-Alexander-Universität Erlangen-Nuremberg. Currently, this lab has about 50 co-workers in the field of machine learning and signal analysis for wearable computing systems with a focus on sports and healthcare.
Available also on Spotify and Apple Podcast
Click here for Spotify
Click here for Apple Podcast
Transcript
Guest: Prof. Dr. Björn Eskofier, Head of the Chair Department Artificial Intelligence in Biomedical Engineering (AIBE), Machine Learning and Data Analytics (MaD) Lab, Friedrich-Alexander-Universität Erlangen-Nürnberg
Moderator: Thomas Reinhardt, Director Corporate Campaigns & Customer Communication, Infineon
Date of publication: 23 February 2023
***
Moderator:
Hi everyone. Welcome to a new episode of the #MakeIoTwork podcast. My name is Thomas Reinhardt, I am your host, and I am excited to have this great opportunity sharing this podcast with all of you.
There has been a lot of discussion about the importance of good health and well-being, especially as the world population rises and at the same time life expectancy is increasing. The fast-growing cost of acute care are pushing the healthcare systems worldwide to a limit. So, healthcare increasingly places great emphasis on enhancing well-being to prevent illness rather than sporadically treating acute illnesses. The IoT has great potential to support good health and well-being. With smart electronic devices, either worn on the body or even installed at home, it is possible to measure people's fitness and health status and trigger appropriate actions, if required - be it a recommendation to open a window for more fresh air, an indication of a necessary doctor's appointment or even an automated emergency call in the event of a fall. Data mining, algorithms, machine learning and artificial intelligence play a central role in future developments in the healthcare sector so these topics have received a lot of attention in medical research recently. This is what I want to talk about with my guest today. Professor Björn Eskofier is heading the Machine Learning and Data Analytics Lab of the FAU. Currently, this lab has about 50 co-workers in the field of machine learning and signal analysis for wearable computing systems with a focus on sports and healthcare. Professor Eskofier, thank you very much for being our guest today.
Guest:
Yes, the pleasure is mine. Thank you very much for inviting me.
Moderator:
As I said in the introduction, the healthcare system is facing major challenges. As consumers, we are being urged to take greater responsibility for our very own health. Healthcare is self-care, so to speak. What other developments can we observe in the digital healthcare system?
Guest:
Yes, thank you very much for the question. There is a lot of things that are currently being done in digital healthcare, and it's really exciting to be part of this journey internationally. So, I think there's three major developments that we currently see. There is an increasing use of digital apps and smartphone wearables that are used to collect data. There is also better ways and research on how to extract and use health data, because you have data in various silos that are not necessarily connected. And then thirdly, and that's of course, the area in which most of my labs work is being done, you have artificial intelligence and machine learning algorithms that make use of the data. So, the combination of collecting data, linking data, and then using data with artificial intelligence and machine learning, that's I think the three important and interesting directions that we currently see in digital healthcare.
Moderator:
It sounds to me as if health data, its extraction and its use in the sense of healthcare and the prevention of diseases, are the top priority. What developments can we observe here in the area of research?
Guest:
Well, there are so many things that we could talk about. So, I would probably just touch base on the main research topics that we currently do in my lab at Friedrich-Alexander-University in Erlangen in Nurnberg. But internationally, you could of course, name a lot of development. So, for example, internationally and beyond what my lab is doing, there is automatic interpretation of medical images as one of interesting areas.
There is genome and proteome and in general omics information that is used off of humans to interpret their future health states. And there's all kinds of prediction algorithms for different diseases in neurology and oncology in neurology and whatnot that are used to predict future health events and the top interventions that you could do with a certain patient. But in our own line of research, we are doing things that are centered on taking human bio signals. So, these might emerge from digital health devices. So, for example, wearables that are worn on body, but also cameras and night vision cameras and other ambient sensing devices that give us information about the status of the human body in various dimensions, like, for example, regarding heartbeat information, electrocardiogram information, breathing information, and other similar things.
And we're making use of these data. So, these are subsequently analyzed, but I think we'll come to that also later. But we're also developing novel sensing concepts. So not only the traditional sensing concepts that I've touched base on so far, so using inertial measurement unit systems and electrodes to measure bio signals and cameras, RGB video cameras to collect information about humans and possibly their health status. But we're also developing, as I said, novel sensing concepts. And these are, I think, very exciting. So, I'm part of a “Sonderforschungsbereich” in German or “Collaborative Research Center” in English. So that's a 35 million - 12-year endeavor financed by the German Research Foundation, where I, together with other researchers, so about 20 PIs (principal investigators) are researching into how to use radar and other RF wave-based detection systems to generate also information about the health status of the human. So, we call this Imparto-Kinesthetic sensing technology because we are, so to say, looking into a human with these sensing technologies and derive information about their kinesthetic sensing so, body pose and also the facial expression to understand better what the health status of an individual is, for example, with respect to Parkinson's disease or with respect to depression, to say something about mental health.
Moderator:
That’s very interesting and I already get the feeling that you have a wide variety of projects and topics that you are covering. And you also talked about collaboration. That’s the point where I would like to better understand how, in those kinds of projects, the collaboration between research – academic institutes – on the one hand, and businesses on the other hand, can work together. What is important for the success of such a large project?
Guest:
Yeah, I think that only teams of multiple disciplines can solve the complicated challenges in healthcare. I mean, a lot of the people that are listening to this podcast will probably know something about how complicated also the digitalization in healthcare is and how many challenges we face. So, I think that only teams of engineers, medical experts, industry representatives and entrepreneurs can really implement changes in digital healthcare. In concrete measures that means that my team and you've already said that I have about 50 researchers in my lab that, so to say, directly are supervised by myself and by my team of postdoctoral and assistant professors. So those people get very often the push from my side to also spend time with their collaboration partners.
So that means that if I supervise a machine learning engineer that comes up with novel interpretation algorithms for bio signals that are collected in a certain disease area, like, for example, Parkinson's disease, then I challenge these people to spend time, for example, with the medical experts. So, they need to be also in the university hospital, spend time with their medical counterparts, but also with patients to understand their needs and to also understand where technology is really most helping with the digital tools, the patients of our age. So, it's not only about coming up with a solution, but it's also about coming up with a solution that actually helps the patient and the medical expert in daily routine. And then, of course, you're an industry representative that I'm talking to. So, this is also a very important piece of the puzzle.
So, we work a lot with industry, both small/medium enterprises, large industry, as well as startups. So my lab has also co-founded a couple of startups that actually bring this technology to the market. Because very often you see great, fantastic research results that work very well in lab collective data sets. But then if you want to bring them into real clinical care, especially in the world of medicine, they fail because they have been trained as a machine learning algorithm with certain data in the lab and then in the wild, as we call it. So, in the real world they often see things that they have not been well prepared for and therefore the solutions that work super well in research and in the lab don't work in the real life. So, working with industry, working with startups is for me such an important piece of our work because that really ensures that we not only research for the purpose of doing something interesting in science, but that we actually are also changing the lives of patients and medical experts alike.
Moderator:
That’s a very good point. And maybe you have one or two concrete examples where you can illustrate, what comes out of such a research project?
Guest:
Yeah, sure. So, I think two things that the lab is currently still doing and which I find personally also super interesting for various reasons, is our work in neurology and in women's health. So, in neurology, my lab is contributing for the last 15 years to automated analysis of symptoms and symptom changes in chronic diseases, chronic neurological diseases, like for example, Parkinson's, which I used as an example a couple of times so far, I have a personal story with Parkinson. So, my uncle unfortunately died from the disease a couple of years ago and from, well, my time as a young adult, I remember him suffering from the disease and from the symptoms that he was experiencing. So, it was personally also motivating for me as a researcher to contribute to this area.
And you really start small. You start with simple sensors that you use together with medical experts in real patient cohorts and then you make a lot of mistakes. You do a lot of things that are okay in science and research. You test things and certain things, certain sensing concepts, certain algorithmic concepts don't work, but some do work. And so, over the years we have developed one of the, I think worldwide largest databases in Parkinson's disease symptom tracking. So together with our university hospital and with many national and international partners, we are quite proud to have, as I said, one of the largest or probably the largest database regarding movement recording in Parkinson's disease. And with this database, which is of course an important prerequisite for any AI and machine learning, probably most of the listeners of this podcast know that any machine learning system can only be as good as the data that use to train it. You can now in 2023, so after 15 years of research and after collecting a lot of data detect and also predict the health status of a Parkinson patient quite well. And we have transferred this knowledge into a startup. So, the startup is currently just at the brink of hopefully becoming getting a marketable product onto the at least German healthcare market. So, we are in the process of getting a DiGa registered. I think this is quite specific but also very interesting. So, listeners that are not familiar with this concept might look into that. It's called the “Digitale Gesundheitsapplikation” (DiGa), and the interesting thing is that currently only Germany has it.
It created a lot of buzz on the German healthcare startup market because if you have a registered DiGa, a medical expert can prescribe your app on recipe, meaning that you get quite directly into Reimbursement. And that's of course for a startup that doesn't have the time to wait for years until you have proven that your system works, a quite attractive business concept. So here I'm really happy that my lab and myself contributed to hopefully a system that can help patients in Parkinson's disease in their daily symptom detection and then also in self-support and then also in supporting the treatment of a patient him or herself. And it was quite an interesting journey to follow that along over the last 15 years.
Moderator:
Thank you very much for the two really lively examples. That was very interesting. But you also mentioned one point and that comes to my mind. You said, of course, with regard to machine learning, the data that is generated, processed and stored, I have to ask: What about the protection of this very sensitive data, as it is fitness and personal health data?
Guest:
No, of course, now I was touching base on neurology and one specific example, Parkinson's disease. But I've also mentioned women's health. So, in women's health we are currently also involved in two large projects and also these projects. So, both Parkinson's disease, neurology as well as women's health only work in this collaboration that I've mentioned. So, there's of course medical experts involved, there is national and international partners involved that I can't all mention here. But in women's health, we are part of my lab and myself, we are part of two large projects. One is funded by the German Ministry of Healthcare and this is centered on digital support of pregnant women. So, to make the complete care of a person that is pregnant, of course, a woman that is pregnant, supported by digital tools. So, something that you can concretely do here is, for example, bringing the tracker that detects the fetal ECG. So, the hard function of the unborn baby to a mother's home. So instead of going to the doctor, to the medical expert and having your fetal ECG measured there, you can already do this at home. And this is things that we do research on, to bring a digital care concept for pregnant women to Germany. And this is of course still research, but we are really hoping that in a couple of years the first startups are using these kinds of ideas and make products out of it and then also improve and digitize the process of digital care in pregnancy.
Yeah, that is of course a super important point and especially living in Europe with the values that we have in the European Union, this is something that is that center in all our improvements and all our research. But I'm really happy that the European Union has also these values put forward in a lot of its legislation. So, interestingly, I was a visiting researcher at MIT, so, Massachusetts Institute of Technology in Cambridge, Massachusetts in 2018 when GDPR, the General Data Protection Regulations of the European Union were put into practice. And the European Union was a little bit like, well seen as, okay, why are they doing all these regulations from the outside? The host that I had at MIT was actually saying that this is a great thing because it defines who data really belongs to, and that's the individual person. That's, of course, a very simple thought, but the European Union put this into a law, and that defines also who can control data. And this is, of course, now something that we see very often in having to consent to cookies whenever we browse the Internet. So rather, something annoying, but in its core principle, it's a good idea. Now the question is how we transport this to healthcare. Because in healthcare, if you just imagine your own situation, you probably had some medical diagnostics in the past. So, for example, you went to the university hospital to get a medical image, and then subsequently, you go to your general practitioner to get information about what came out of this medical picture. And the intervention that you get is, of course, based on this medical information that you have.
So now the challenge is, of course, how to transport this into healthcare. Because you might know from your own practice that the last time you got a medical image, for example, in a university hospital, the images then in the university hospital and subsequently you go to a general practitioner to get information about what came out of this image that was taken off of you. But you never have the medical image yourself. Now, you might not be interested in that, but we as researchers are very much interested in all these data. So, if I want to build a great machine learning system, I want to have data from thousands of patients. And that was exactly the argument that I made. So, in our Parkinson's disease research and our neurology research, we had to collect data over 15 years. And that's of course also a challenge to innovation because if I have a certain question that I would like to answer with large amounts of data, I always have to or very often have to collect these data prospectively for a certain study purpose. But these data are mostly all available, but they are in their silos. So, the data are with the university hospitals, with the general petitioners, a little bit with ourselves and all those smart wearables that we are carrying around. But nevertheless, the data are dispersed in silos. So, I'm very motivated that I'm part of another endeavor by the European Union that currently designs the so-called European health data space, or in short, EHDS. So, the EHDS is another legislation like the GDPR that defines that all medical data should reside with the patient, him or herself, and so that the patient can control those data. So, for example, a patient of the future, once this is put into practice, can give donate the last medical image to research, because this is GDPR compliant and it also works in this European health data space ecosystem. And now, importantly, because you asked for it, this makes control over the data. So, protection of this data a central key asset of the whole puzzle, because now a patient sees what data is used really for what purpose and can also allow certain usage of data and disallow certain usage of data.
Moderator:
That’s a very interesting development. Thank you very much for the insights. And unluckily, we already have come to the very last question of this podcast. And it always revolves around the more distant future, I would say. So, I would like to ask you to take a look into the crystal ball. So what exciting new applications and devices can we expect in the area of smart healthcare? Where will the journey take us?
Guest:
Wow. That's a very broad question and I think very challenging to answer because we all know that predictions, especially if they are about the future, are very hard to make. But I'll try myself. So, what we currently see in the not too distant future is, of course this for me, very interesting area of RF, radio frequency wave based, radar-based sensing. So, there's really a lot of interesting insights that we can generate from these kinds of non-invasive and touch free data collection systems. So, for example, we are currently doing a study at the end of lifetime. So, in palliative care with radar systems that measure heartbeat information and that measure breathing information. And with these systems, we get information about the last phase and the last days and actually hours of life. And this is first of all a data collection study. But with the data, we hope that we can also come up with biomarkers that really tell us predictively when these last days and last hours of life are really arriving. So that you can actually stop palliative care or change palliative care and inform the relatives at the right point in time, that it's now well time to unfortunately say goodbye to your relatives. So, these are things that I think are a little bit closer on the horizon, a little bit farther away, are things that we just do a little bit of research with currently in my direct vicinity and that's brain computer interfaces. That's the technology that I'm really fascinated about because currently the world is talking about artificial intelligence systems and chat GPT and in general, AI systems that can replace human function. I think the machine learning experts like myself and also much better-known international experts like Joshua Bengio and Jeffrey Hinton and other very well-known people in the area of artificial intelligence. They predict that, well, until the AI is replacing us, human intelligences, that will take some 40 or 50 years because the systems are just not there yet. But that's, I think, a more philosophical discussion. But what could really be interesting if we combine the technical intelligence and the biological intelligences even better by, as I said, brain computer interfacing. And there are really interesting developments that might in the next 20 or 30 years actually lead to interesting developments in, well, combining the advantages of technical systems and of biological intelligences. And then of course, there's a lot of interesting developments also on restoring body functions. So, for example, having even better restoration of hearing function, of vision function by the combination as a set of technical devices with the biological intelligence.
Now, how the world will look like in 100 to 150 years or even beyond that, I do not dare imagine. Maybe we will be able to upload ourselves into the cloud with the appropriate brain computer interface. But I think I'll leave the speculation here to the listeners and let you conclude the podcast.
Moderator:
Thank you very much for the insights of the today’s topics and also the glimpse into the future. Thank you very much, Professor Eskofier, for your exciting insights.
This brings us to the end of this episode. Dear listeners: If you want to learn more about us and our world of IoT, visit our website www.infineon.io. If you're currently listening to us on Spotify or Apple Podcast, we'd love for you to subscribe to our podcast and leave a little review. Now it only remains for me to wish you a good time. Take care and see you next time.